Probability (of success) is the chance of an event happening For example, there might be an 80% chance of rain today Odds are the probability of success (80% chance of rain) divided by the probability of failure (% chance of norain) = 08/02 = 4, or 4 to 1 Logodds is simply the logarithm of odds 1• Ordinal logistic regression (Cumulative logit modeling) • Proportion odds assumption • Multinomial logistic regression • Independence of irrelevant alternatives, Discrete choice models Although there are some differences in terms of interpretation of parameter estimates, the essential ideas are similar to binomial logistic regression2) At least how many
Simple Logistic Regression
Odds vs probability logistic regression
Odds vs probability logistic regression-Definition of the logistic function An explanation of logistic regression can begin with an explanation of the standard logistic functionThe logistic function is a sigmoid function, which takes any real input , and outputs a value between zero and one For the logit, this is interpreted as taking input logodds and having output probabilityThe standard logistic function → (,) isLogistic regression models a relationship between predictor variables and a categorical response variable For example, we could use logistic regression to model the relationship between various measurements of a manufactured specimen (such as dimensions and chemical composition) to predict if a crack greater than 10 mils will occur (a binary variable either yes or no)



Q Tbn And9gctxz8owky Sul84xtk4ggzacxwhkmhguhlxwyjj9avufagdrhwm Usqp Cau
The coefficient returned by a logistic regression in r is a logit, or the log of the odds To convert logits to odds ratio, you can exponentiate it, as you've done above To convert logits to probabilities, you can use the function exp (logit)/ (1exp (logit)) However, there are some things to note about this procedure For a primer on proportionalodds logistic regression, see our post, Fitting and Interpreting a Proportional Odds Model In this post we demonstrate how to visualize a proportionalodds model in R To begin, we load the effects package The effects package provides functions for visualizing regression modelsLast class we discussed how to determine the association between two categorical variables (odds ratio, risk ratio, chisquare/Fisher test) Suppose we want to explore a situation in which the dependent variable is dichotomous (1/0, yes/no, case/control) and
Why do we need logistic regression Logistic regression predicts the probability of success A success vs failure can take a form of 1 vs 0, YES vs NO or TRUE vs FALSE While the success is always measured in only two (binary) values, either success or failure, the probability of success can take any value from 0 to 1 The probability of81 Introduction to logistic regression Until now our outcome variable has been continuous But if the outcome variable is binary (0/1, "No"/"Yes"), then we are faced with a classification problem The goal in classification is to create a model capable of classifying the outcome—and, when using the model for prediction, new observations—into one of two categories Odds Odds seems less intuitive It is the ratio of the probability a thing will happen over the probability it won't In the spades example, the probability of drawing a spade is 025 The probability of not drawing a spade is 1 025 So the odds is 025/075 or 13 (or 033 or 1/3 pronounced 1 to 3 odds) Moving back and forth
Help logistic postestimation) So the reported metric of margins is the risk rates of two groups by ivariable, and the output of margins rvariable is the absolute risk difference between two groups However, I want the output of logistic indep I have a question about plotting a probability curve for a logistic regression model that has multiple predictors I'm posted this here on SO because I'm wondering about ggplot2 specific solutions, and creating useful graphics from a logit model in ggplot2 So here is an example = Note Probability ranges from 0 to 1 Odds range from 0 to ∞ Log Odds range from −∞ to ∞ That is why the log odds are used to avoid modeling a variable with a




Logistic Regression Estimates Of The Probability Of Arranged Vs Download Scientific Diagram




Statistics 101 Logistic Regression Probability Odds And Odds Ratio Youtube
Baseline multinomial logistic regression but use the order to interpret and report odds ratios They differ in terms of The log cumulative odds ratio is proportional to the difference (distance) We can compute the probability of being in category j by taking differences between the cumulative probabilities P(Y =j)=P(Y ≤j)−P(Y Probability vs Odds vs Log Odds All these concepts essentially represent the same measure but in different ways In the case of logistic regression, log odds is used We will see the reason why log odds is preferred in logistic regression algorithm Odds (Safety) = 12/72 = 1787 Now get out your calculator, because you'll see how these relate to each other Odds (Accident) = Pr (Accident)/Pr (Safety) = 053/947 Understanding Probability, Odds, and Odds Ratios in Logistic Regression Despite the way the terms are used in common English, odds and probability are not interchangeable



Logistic Regression Essentials In R Articles Sthda



Logistic Regression Wikipedia
In the previous tutorial, you understood about logistic regression and the best fit sigmoid curve Next, discuss Odds and Log Odds Odds The relationship between x and probability is not very intuitive Let's modify the above equation to find an intuitive equation Step1 Calculate the probability of not having blood sugar Step2 WhereIn video two we review / introduce the concepts of basic probability, odds, and the odds ratio and then apply them to a quick logistic regression example Un The dataset of pass/fail in an exam for 5 students is given in the table below If we use Logistic Regression as the classifier and assume the model suggested by the optimizer will become the following for Odds of passing a course $\log (Odds) = 64 2 \times hours$ 1) How to calculate the probability of Pass for the student who studied 33 hours?




Binary Logistic Regression With Odds Ratios Calculated For The Download Table



Simple Logistic Regression
Now we can relate the odds for males and females and the output from the logistic regression The intercept of 1471 is the log odds for males since male is the reference group ( female = 0) Using the odds we calculated above for males, we can confirm this log (23) = 147Thinking about log odds can be confusing, though So using the math described above, we can rewrite the simple logistic regression model to tell us about the odds (or even about probability) Odds = e β0β1*X Using some rules for exponents, we can obtain Odds = (e β0)*(e β1*X) When X equals 0, the second term equals 10 The log odds are modeled as a linear combinations of the predictors and regression coefficients β0 β1xi β 0 β 1 x i The complete model looks like this Logit = ln( p(x) 1−p(x)) =β0 β1xi L o g i t = l n ( p ( x) 1 − p ( x)) = β 0 β 1 x i This equation shows, that the linear combination models the Logit and model coefficients




Logit Wikipedia
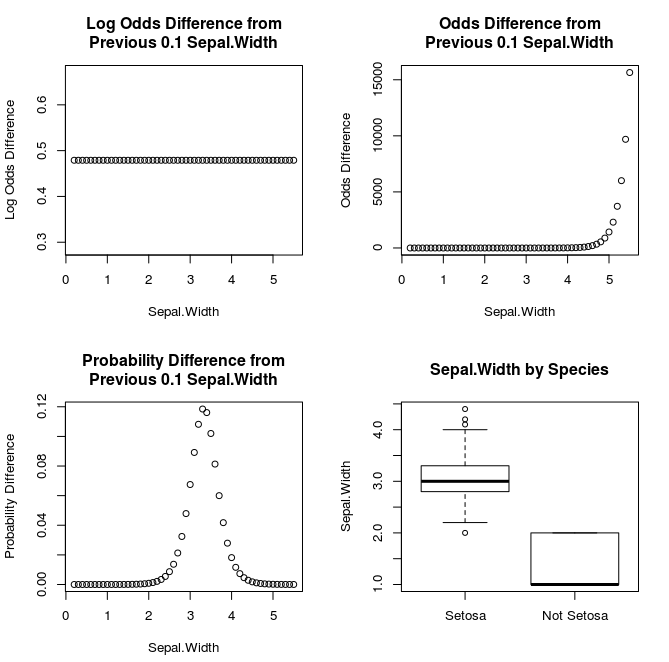



Why Saying A One Unit Increase Doesn T Work In Logistic Regression Learn By Marketing
Marginal Effects vs Odds Ratios Models of binary dependent variables often are estimated using logistic regression or probit models, but the estimated coefficients (or exponentiated coefficients expressed as odds ratios) are often difficult to interpret from a practical standpoint Empirical economic research often reports 'marginal effects Odds vs Probability Before diving into the nitty gritty of Logistic Regression, it's important that we understand the difference between probability and odds Odds are calculated by taking the number of events where something happened and dividing by the number events where that same something didn't happen Tom the reported metric is the predicted probability of a positive outcome (see help margins;
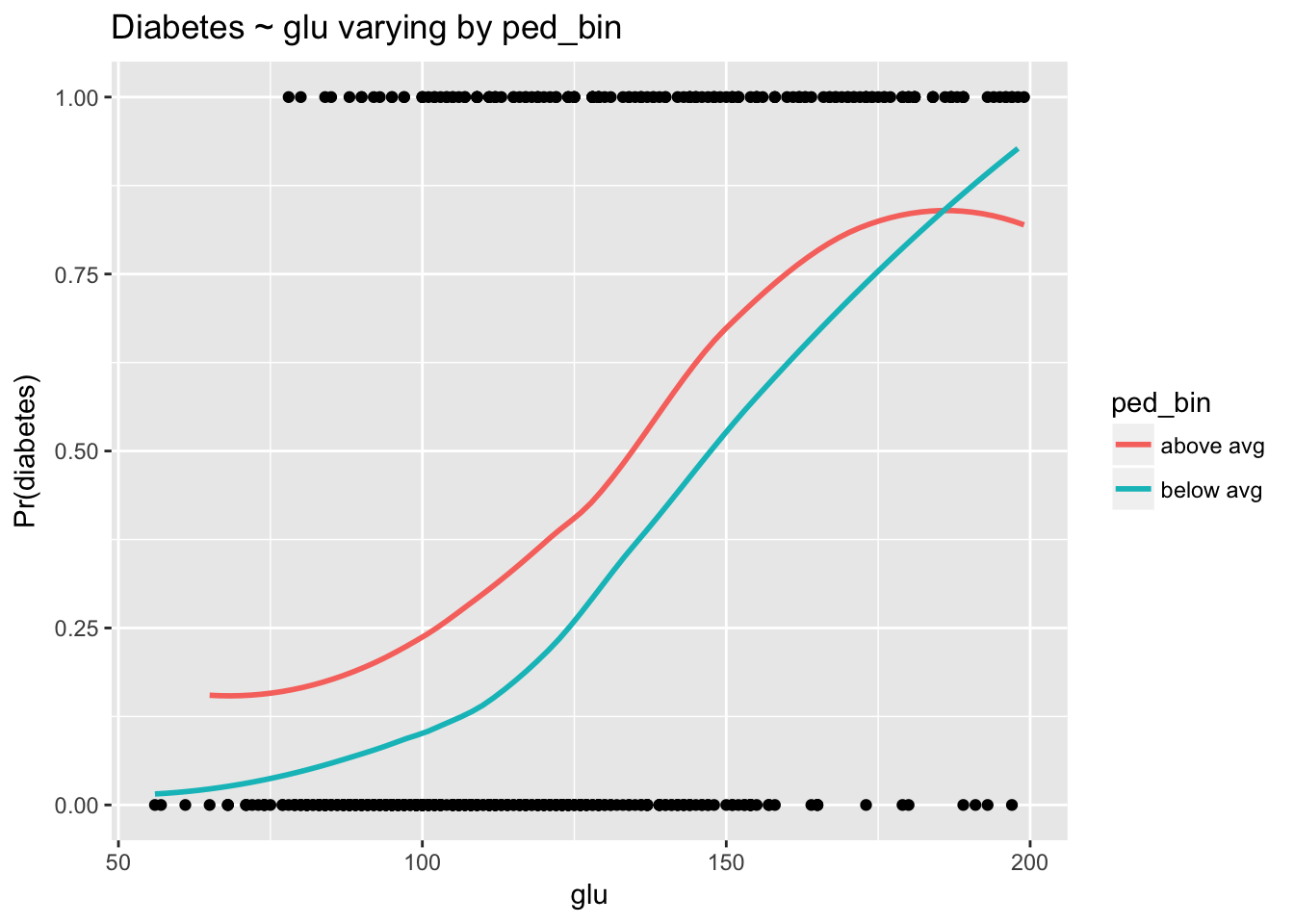



Course Notes For Is 64 Statistics And Predictive Analytics
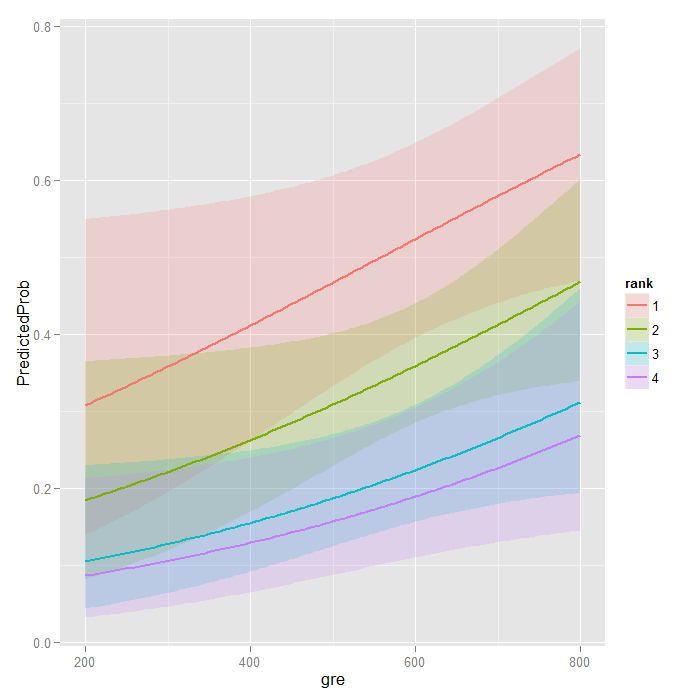



Logit Regression R Data Analysis Examples
An important property of odds ratios is that they are constant It does not matter what values the other independent variables take on For instance, say you estimate the following logistic regression model 1685 x 1 0039 x 2 The effect of the odds of a 1unit increase in x 1 is exp(1685) = 118 Meaning the odds increase by 18%This video explains how the linear combination of the regression coefficients and the independent variables can be interpreted as representing the 'log odds'A Difference between probability and odds b logistic command in STATA gives odds ratios c logit command in STATA gives estimates d difficulties interpreting main effects when the model has interaction terms e use of STATA command to get the odds of the combinations of old_old and endocrinologist visits (1,1, 1,0, 0,1, 0,0) f
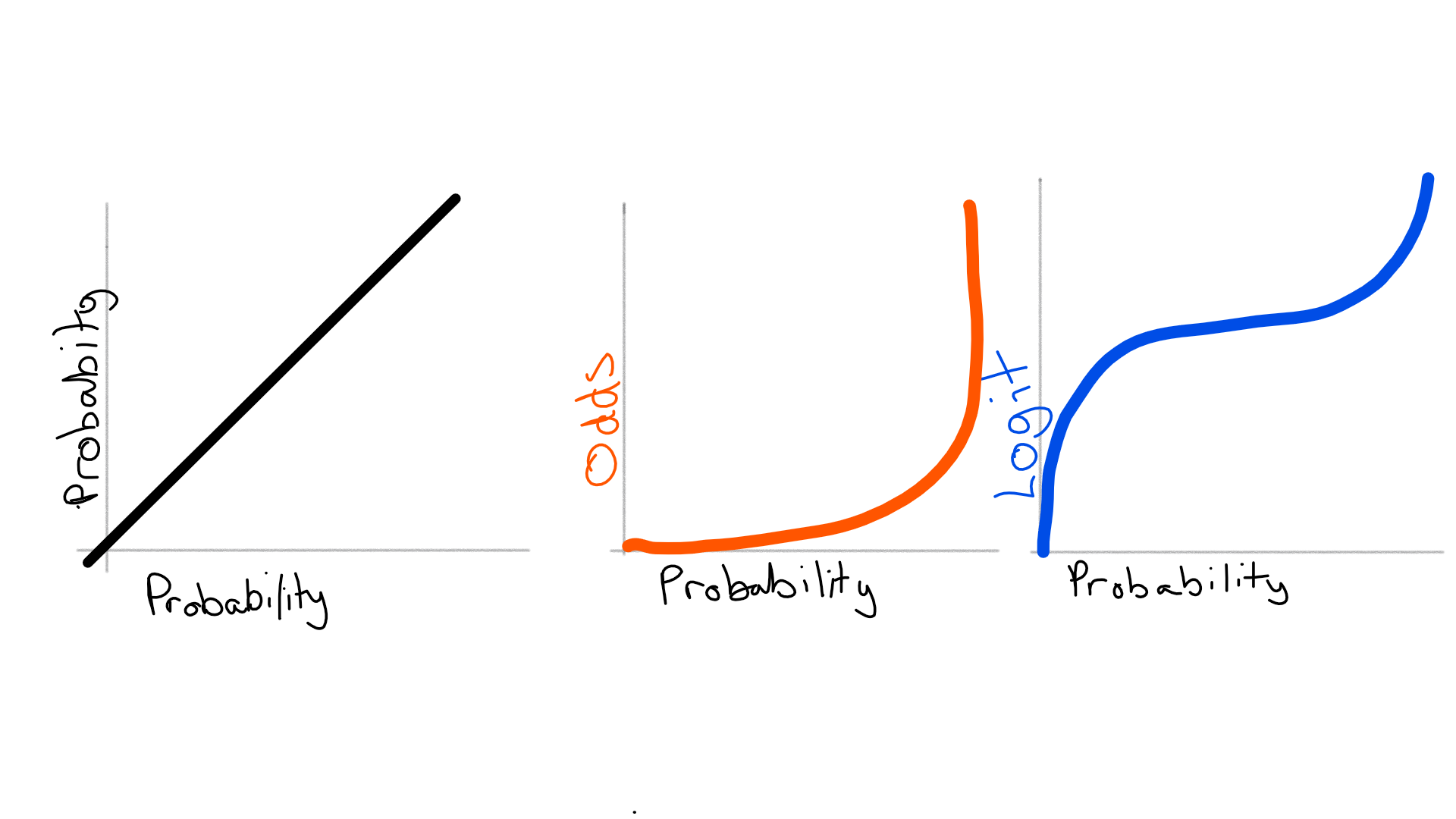



How To Go About Interpreting Regression Cofficients




Logistic Regression 1 From Odds To Probability Dr Yury Zablotski
If z represents the output of the linear layer of a model trained with logistic regression, then s i g m o i d ( z) will yield a value (a probability) between 0 and 1 In mathematical terms y ′ = 1 1 e − z where y ′ is the output of the logistic regression model for a particular example z = b w 1 x 1 w 2 x 2 w N x N The probability that an event will occur is the fraction of times you expect to see that event in many trials Probabilities always range between 0 and 1 The odds are defined as the probability that the event will occur divided by the probability that the event will not occur If the probability of an event occurring is Y, then the probability of the event not occurring is 1YUnderstanding Probability, Odds, and Odds Ratios in Logistic Regression Odds ratios are the bane of many data analysts Interpreting them can be like learning a whole new language This webinar recording will go over an example to show how to interpret the odds ratios in binary logistic regression




Logistic Regression Circulation
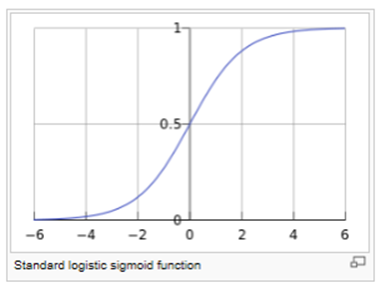



Probability Calculation Using Logistic Regression
Recall that the function of logistic is to predict successful outcomes of that depends upon the the value of other values For mathematical reasons we take the log if this ratio in our estimation process If probability of success is math050/mLogistic Regression and Odds Ratio A Chang 1 Odds Ratio Review Let p1 be the probability of success in row 1 (probability of Brain Tumor in row 1) 1 − p1 is the probability of not success in row 1 (probability of no Brain Tumor in row 1) Odd of getting disease for the people who were exposed to the risk factor ( pˆ1 is an estimate of p1) O = Let p0 be the probability of successHi Arvind, Thanks for A to A In general with any algorithm, coefficient getting assigned to a variable denotes the significance of that particular variable High coefficient value means the variable is playing a major role in deciding the boundar




Chapter 17 Logistic Regression Applied Statistics With R
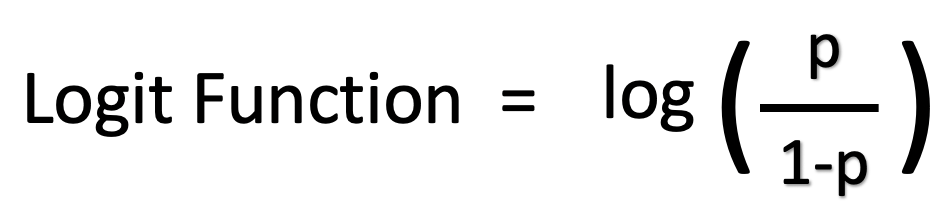



What And Why Of Log Odds What Are Log Odds And Why Are They By Piyush Agarwal Towards Data Science
The interpretation of the weights in logistic re g ression differs from the interpretation of the weights in linear regression, since the outcome in logistic regression is a probability between 0 In a logistic regression model, odds ratio provide a more coherent solution as compared to probabilities Odds ratio represent the constant effect of an independent variable on a dependent variable Here, being constant means that this ratio does not change with a change in the independent (predictor) variableRegression Equation Estimation of Regression Coefficients in Logistic Reg Interpretation Odds vs Logit Obtaining Solutions More Visualizations and Formulas Slide 30 Slide 31 Slide 32 Partial results from Cavenaugh, Giesen, & Steinman (06)
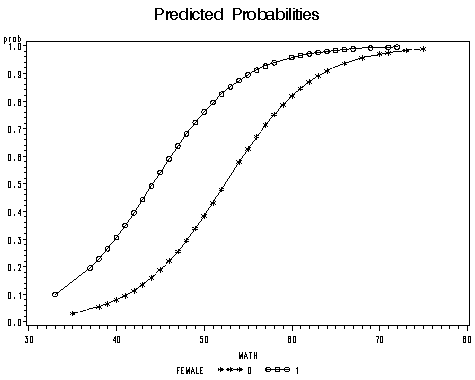



Proc Logistic And Logistic Regression Models




How To Interpret Logistic Regression Coefficients Displayr
There are several types of ordinal logistic regression models Probably the most frequently used in practice is the proportional odds model (Hosmer and Lemeshow, Applied Logistic Regression (2nd ed), p 297) Before we explain a "proportional odds model", let's just jump ahead and do it The logistic regression coefficient indicates how the LOG of the odds ratio changes with a 1unit change in the explanatory variable; The survival probability is if Pclass were zero (intercept) However, you cannot just add the probability of, say Pclass == 1 to survival probability of PClass == 0 to get the survival chance of 1st class passengers Instead, consider that the logistic regression can be interpreted as a normal regression as long as you use logits
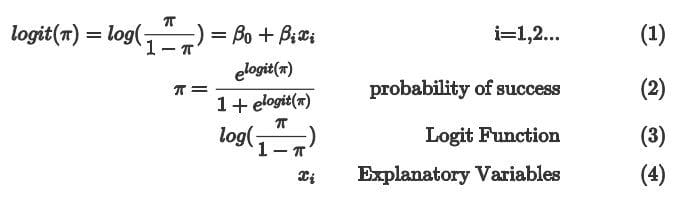



Logistic Regression A Concise Technical Overview Kdnuggets
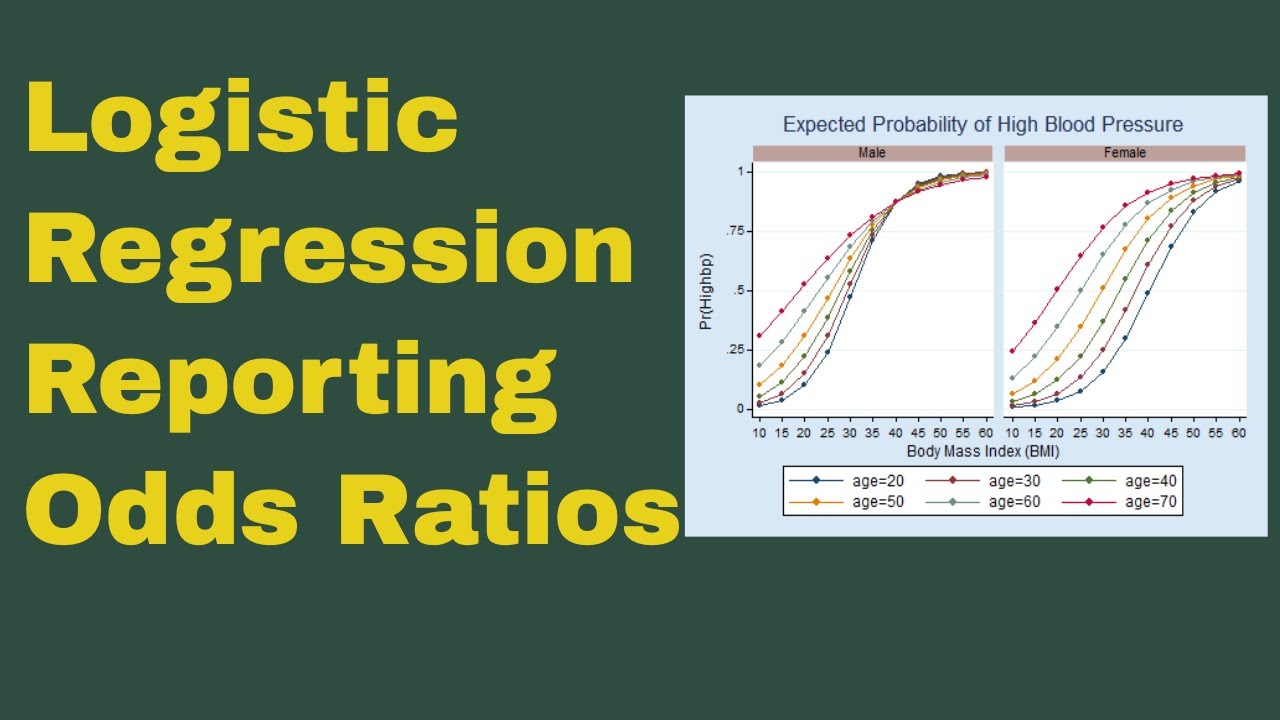



Logistic Regression Reporting Odds Ratios Youtube
The logarithm of an odds can take any positive or negative value Logistic regression is a linear model for the log (odds) This works because the log (odds) can take any positive or negative number, so a linear model won't lead to impossible predictions A logistic regression model provides the 'odds' of an event Remember that, 'odds' are the probability on a different scale Here is the formula If an event has a probability of p, the odds of that event is p/ (1p) Odds are the transformation of the probability Based on this formula, if the probability is 1/2, the 'odds' is 1This is not the same as the change in the (unlogged) odds ratio though the 2 are close when the coefficient is small 2 Your use of the term "likelihood" is quite confusing
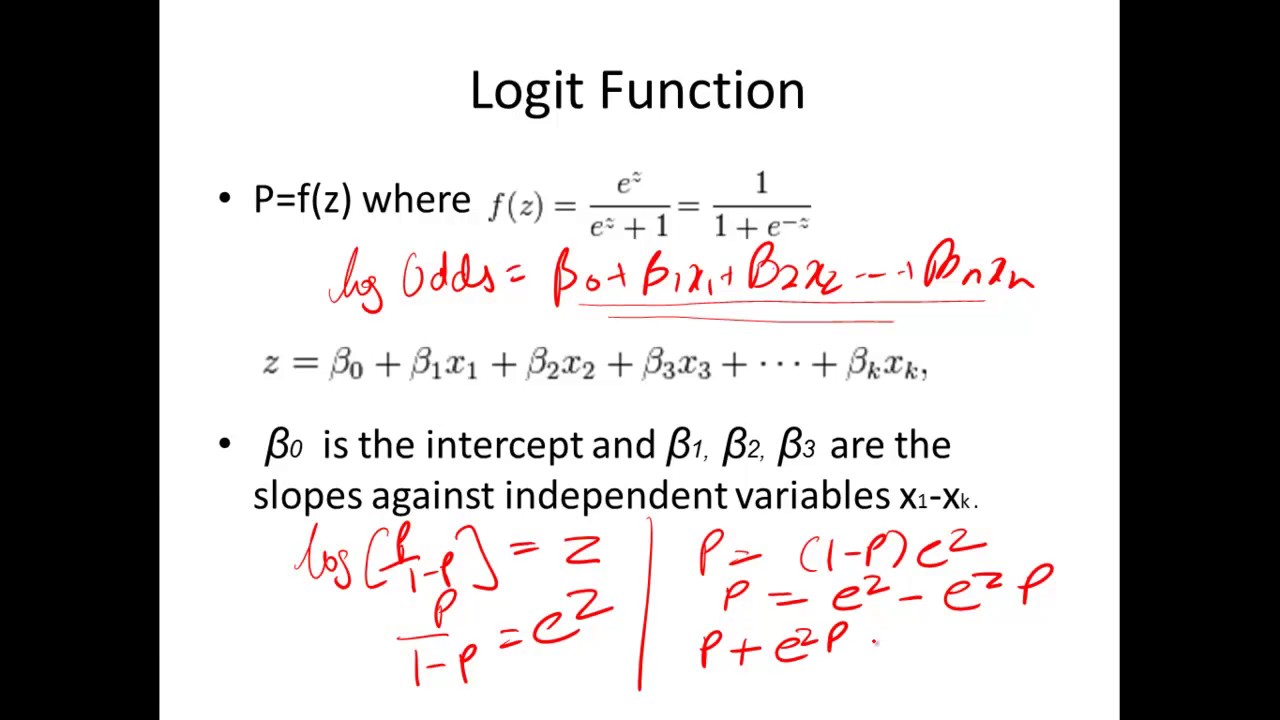



3 Logistic Regression Logit Transformation In Detail Youtube



Logistic Regression
Labs(title ="probability versus odds") 000 025 050 075 100 0 50 100 150 odds p probability versus odds Finally, this is the plot that I think you'llfind most useful because inlogistic regression71 Logistic Regression 711 Why use logistic regression?



Www Jstor Org Stable
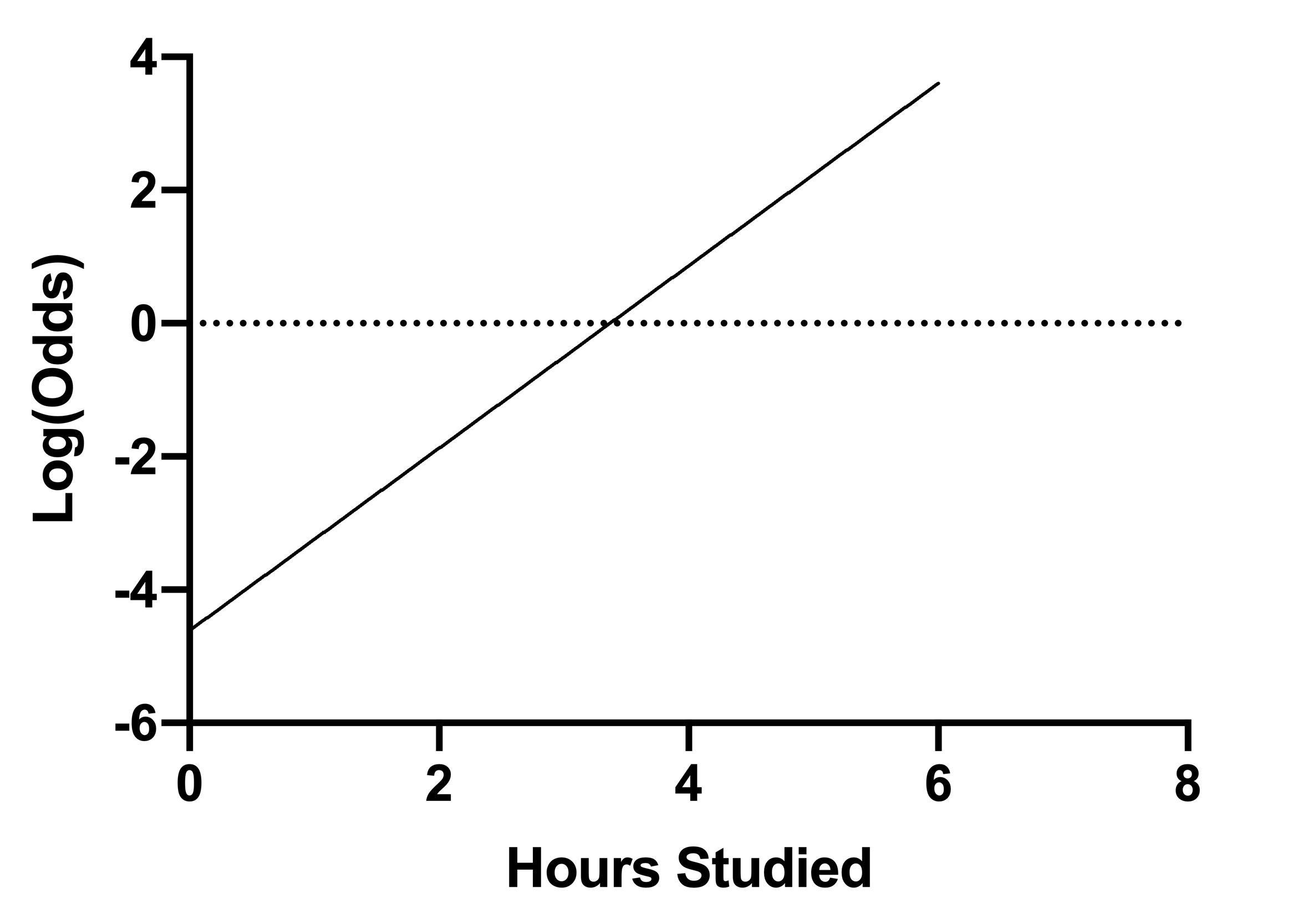



Graphpad Prism 9 Curve Fitting Guide Interpreting The Coefficients Of Logistic Regression
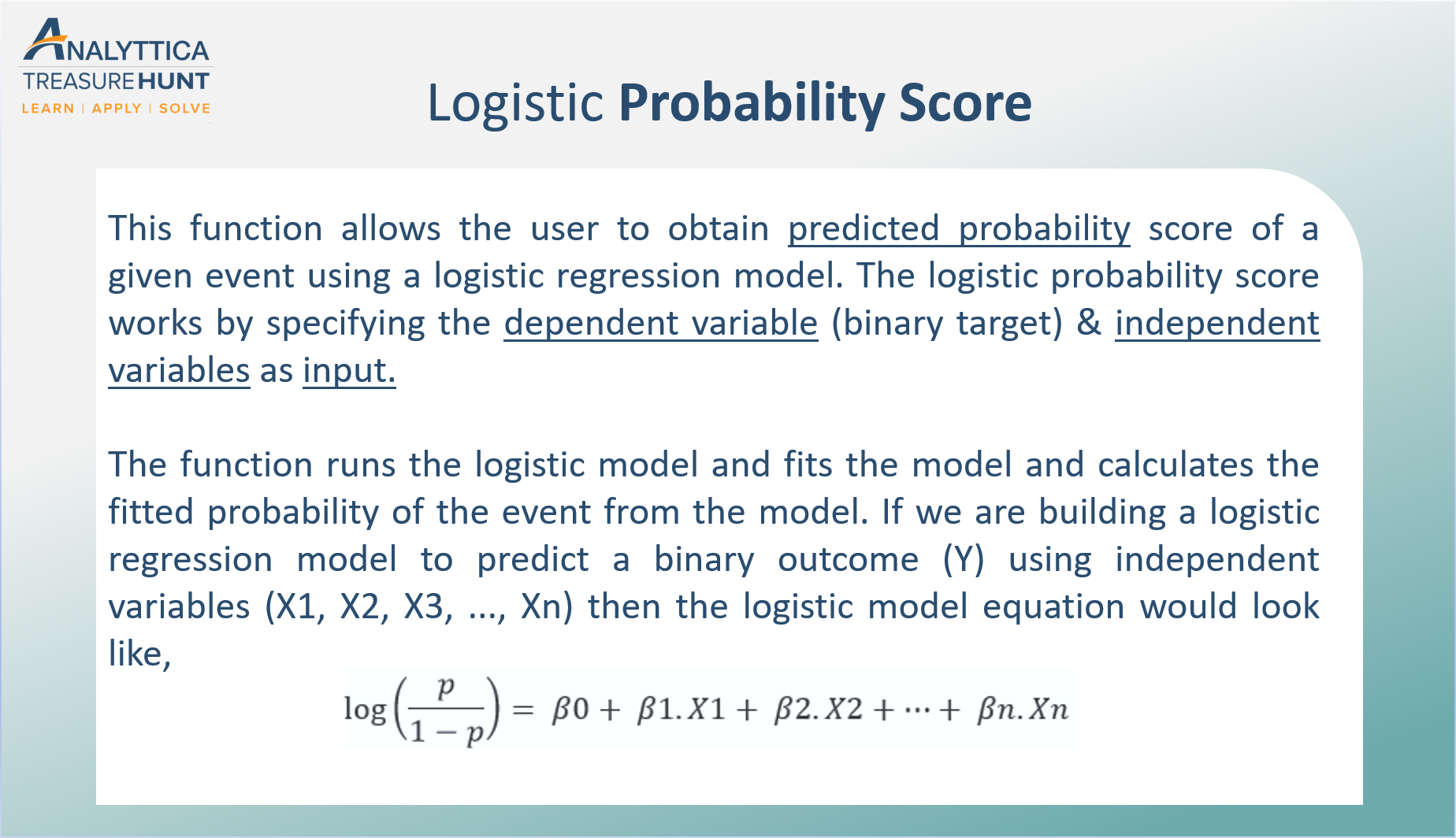



Logistic Probability Score The Logistic Probability Score Function By Analyttica Datalab Medium




Multiple Logistic Regression Analysis



Logistic Regression
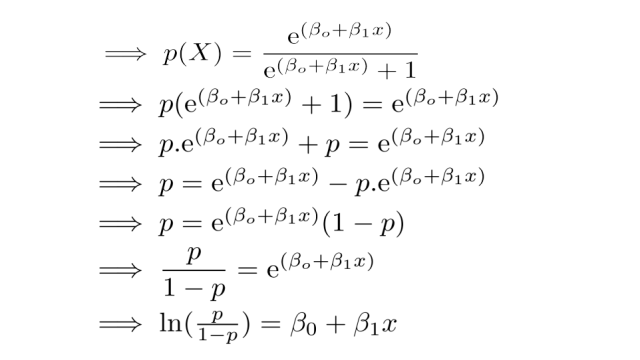



Simplifying Logistic Regression Dzone Ai



1 Logistic Regression Logistic Regression Treats Chegg Com
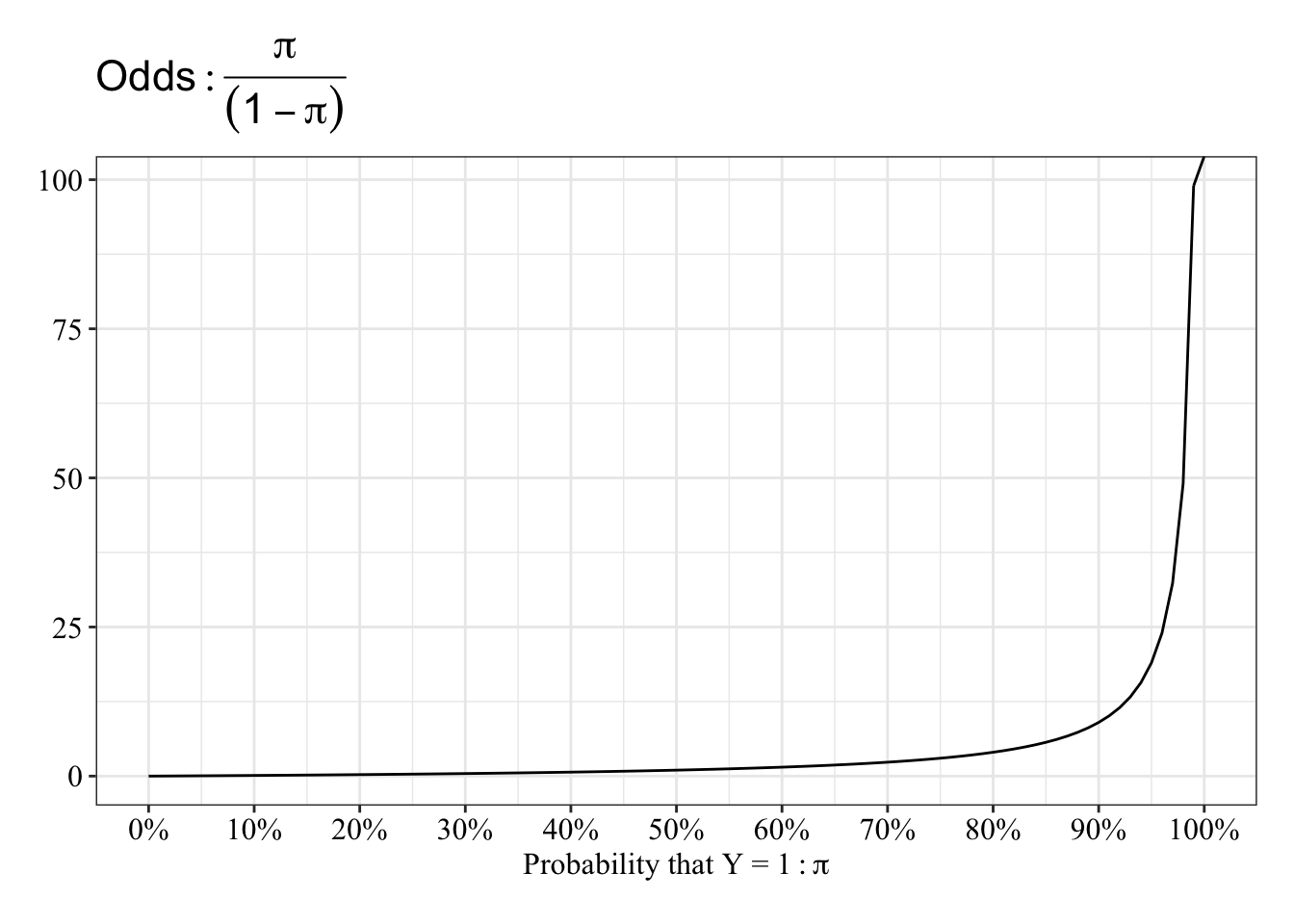



Logistic Regression In R Nicholas M Michalak




Proportional Odds Logistic Regression On Laef The Probability Of Download Scientific Diagram



An Introduction To Logistic Regression
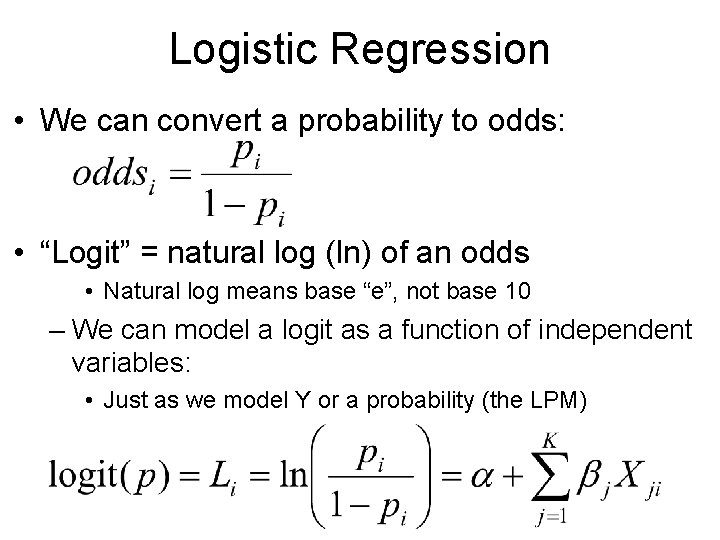



Logistic Regression 2 Sociology 11 Lecture 7 Copyright




What And Why Of Log Odds What Are Log Odds And Why Are They By Piyush Agarwal Towards Data Science



Logistic Regression
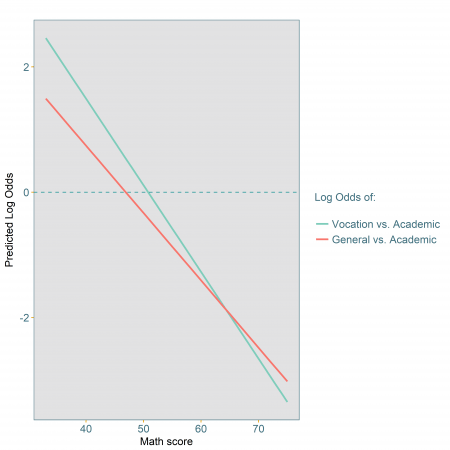



Presenting The Results Of A Multinomial Logistic Regression Model Odds Or Probabilities Select Statistical Consultants
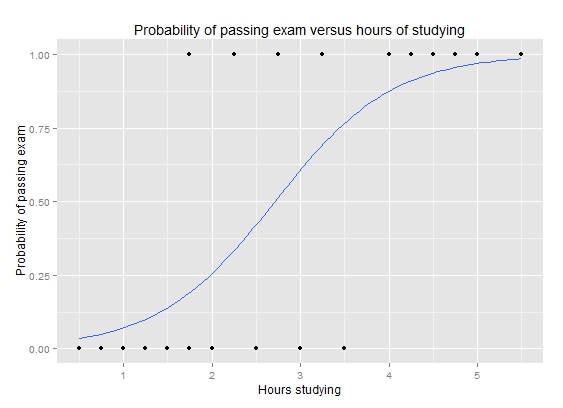



Understanding Logistic Regression Worldsupporter



Relative Risk Ratios And Odds Ratios




Gr S Website
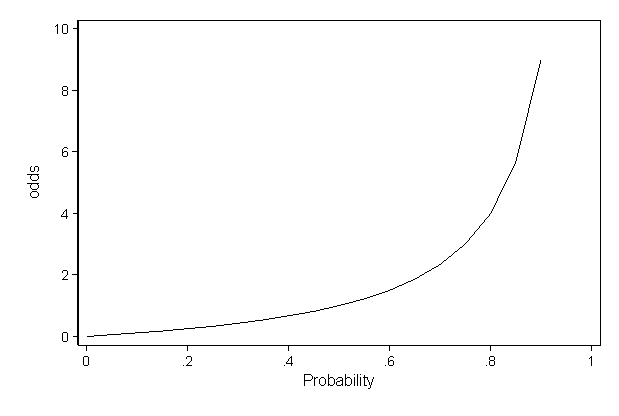



Faq How Do I Interpret Odds Ratios In Logistic Regression



Confidence Intervals For The Odds Ratio In Logistic Regression With Two Binary X S Pdf Confidence Interval Logistic Regression



Logistic Regression Multiple Logistic Odds Ratio Statsdirect




What And Why Of Log Odds What Are Log Odds And Why Are They By Piyush Agarwal Towards Data Science
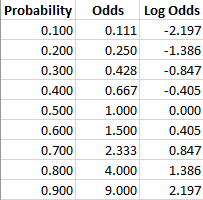



Log Odds Definition And Worked Statistics Problems



Logistic Regression From Bayes Theorem Count Bayesie



Solved Question 2 In Logistic Regression The Probability Chegg Com



1




Percent Change In Odds Ratio For The Multiple Logistic Regression Nas Download Scientific Diagram




R Calculate And Interpret Odds Ratio In Logistic Regression Stack Overflow



Http U Demog Berkeley Edu Andrew Teaching Compare Prob Pdf




Logistic Regression In Python Blog By Cory Maklin
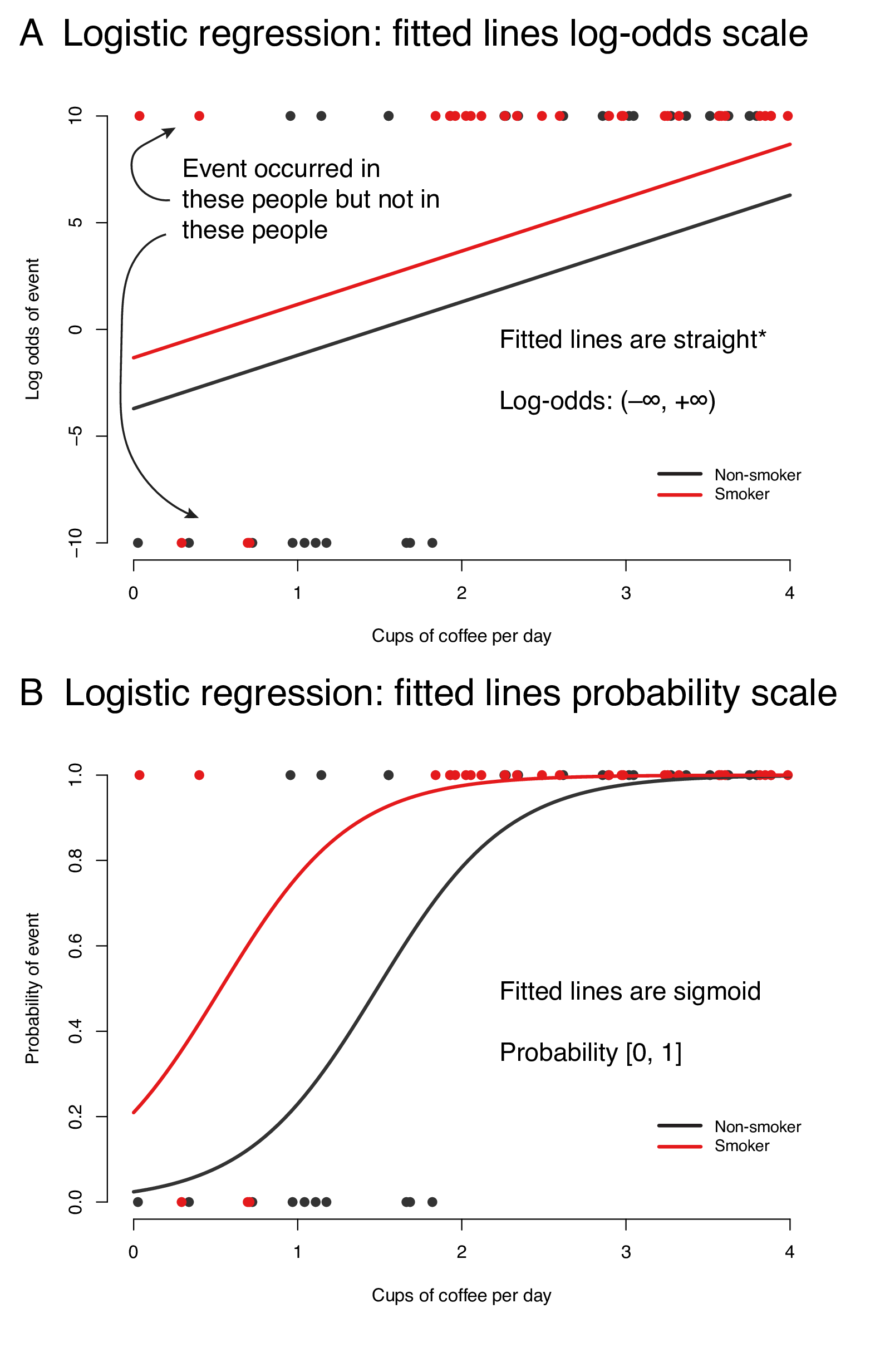



9 2 Binary Logistic Regression R For Health Data Science




Use And Interpret Logistic Regression In Spss
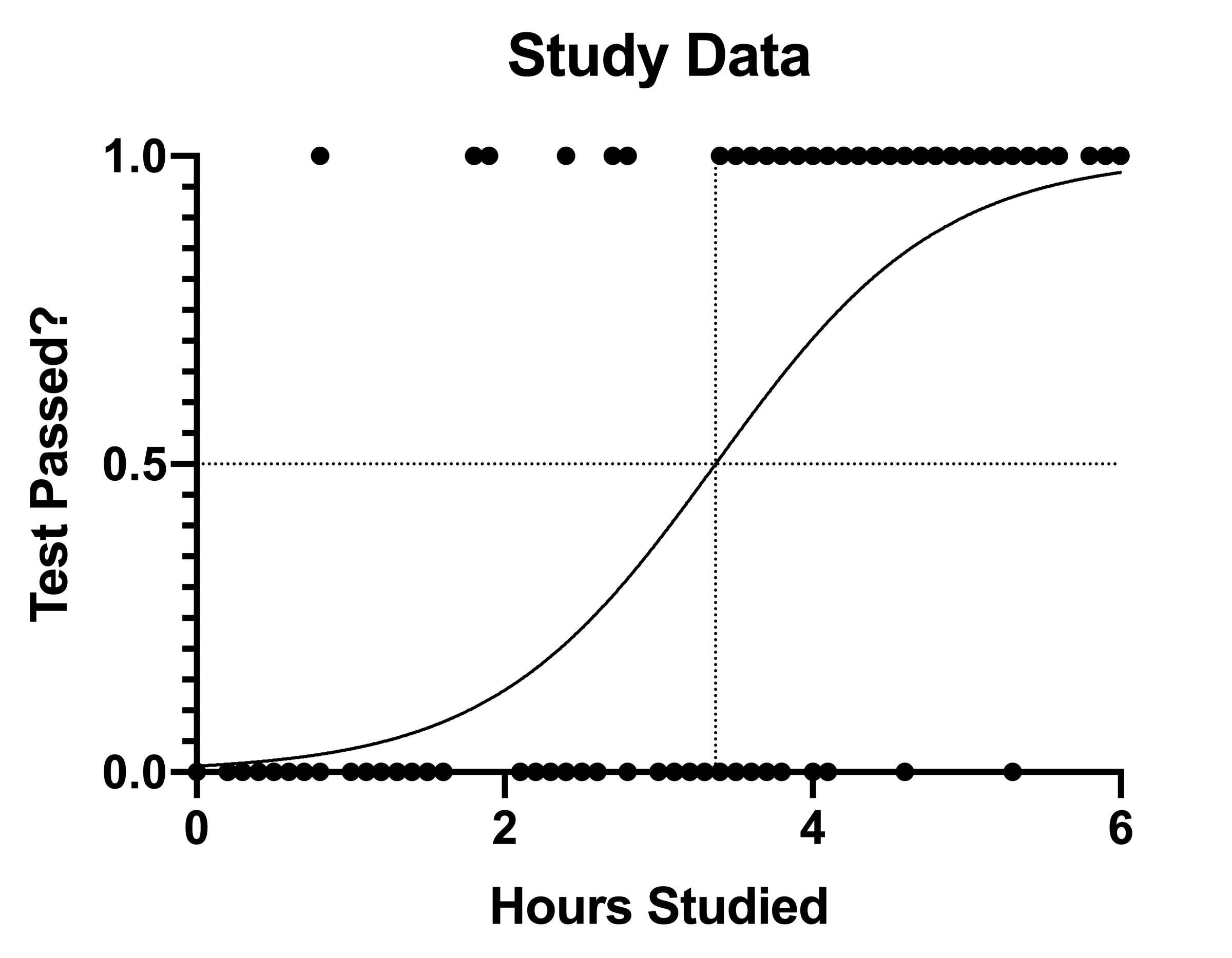



Graphpad Prism 9 Curve Fitting Guide Example Simple Logistic Regression




How To Interpret The Weights In Logistic Regression By Mubarak Bajwa Medium



Logistic Regression Wikipedia




Logistic Regression Data Vedas




Logistic Regression Wikipedia




Logistic Regression Analysis An Overview Sciencedirect Topics
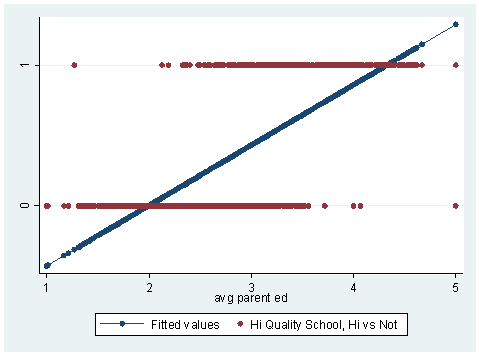



Logistic Regression With Stata Chapter 1 Introduction To Logistic Regression With Stata



Ii Binary Logistic Regression Insecticides Xlsx Chegg Com
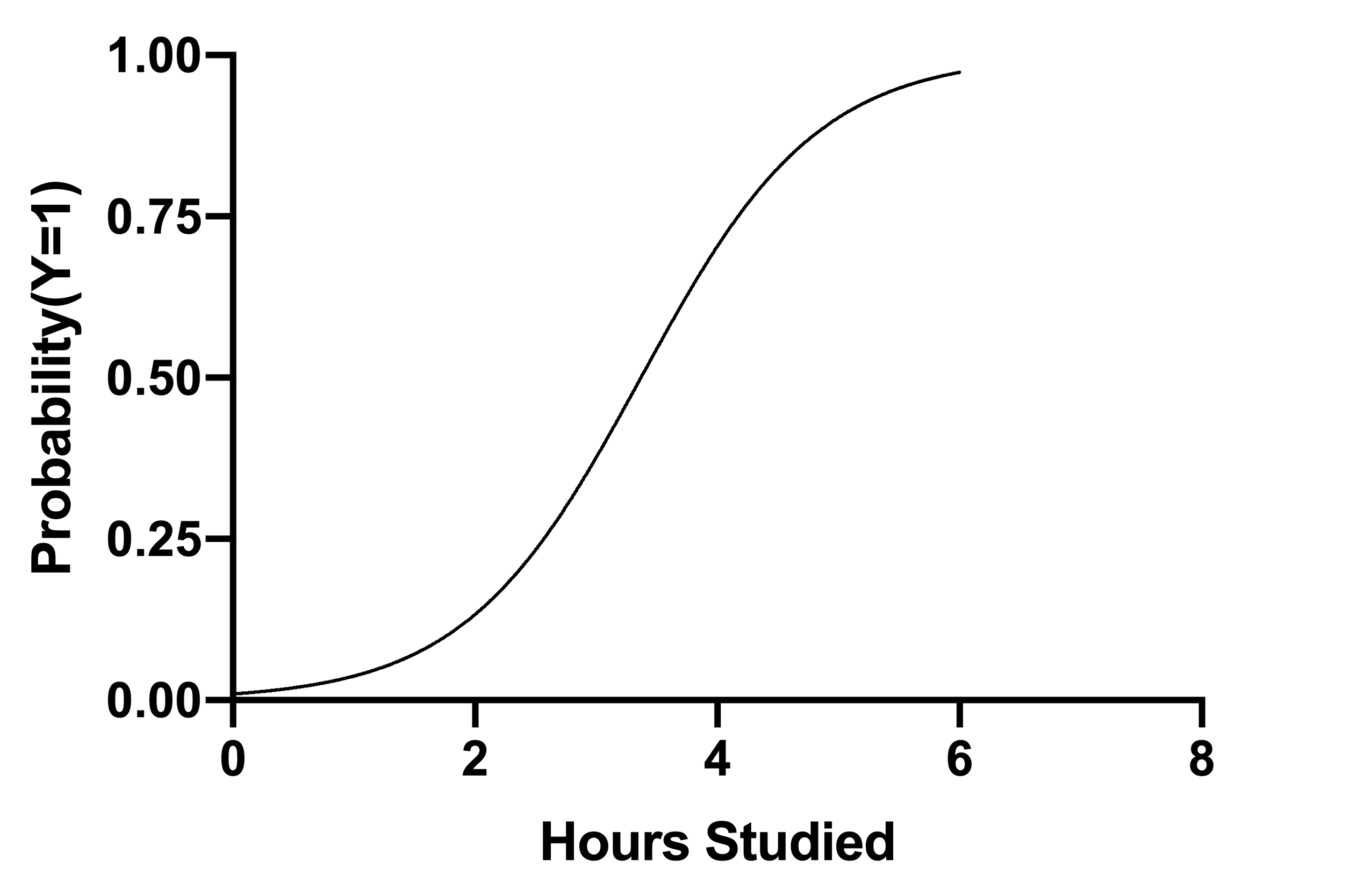



Graphpad Prism 9 Curve Fitting Guide Interpreting The Coefficients Of Logistic Regression




The Difference Between Relative Risk And Odds Ratios The Analysis Factor
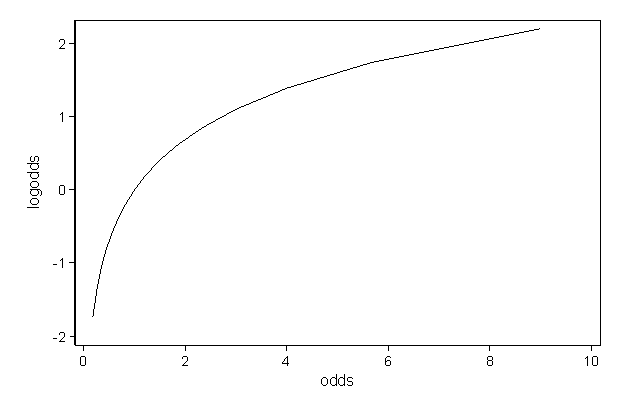



Faq How Do I Interpret Odds Ratios In Logistic Regression
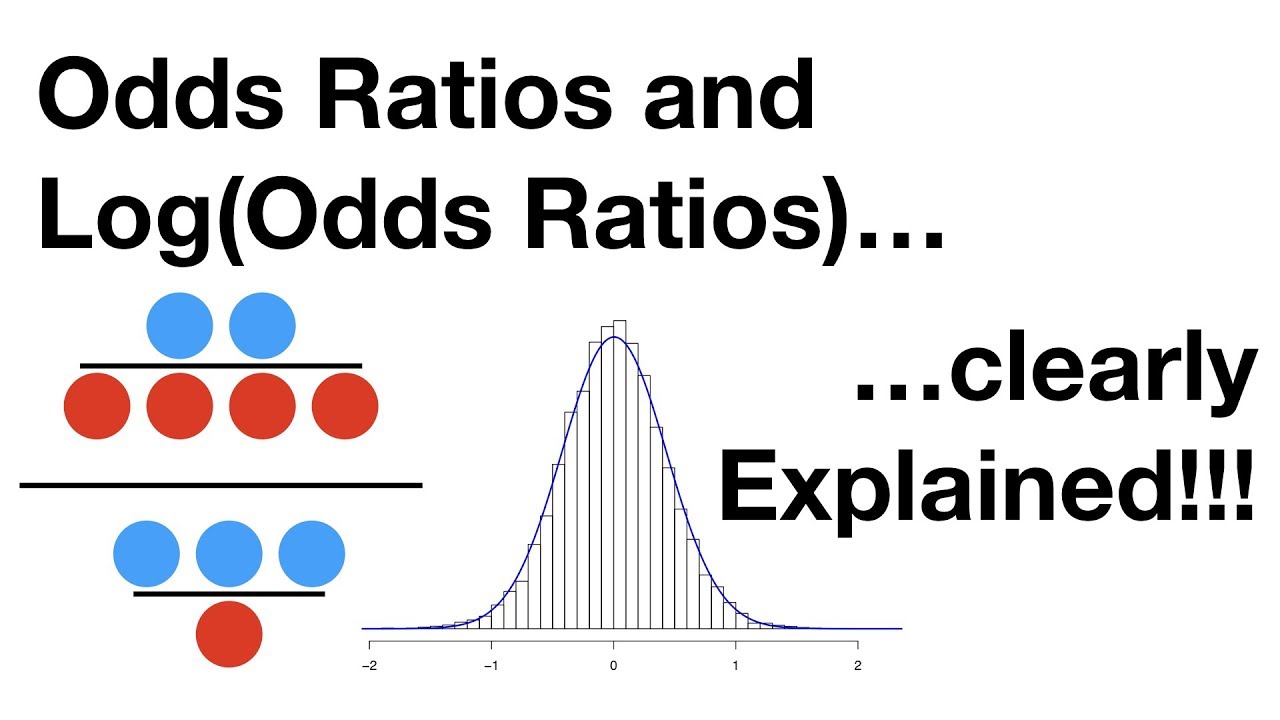



Statquest Odds Ratios And Log Odds Ratios Clearly Explained Youtube
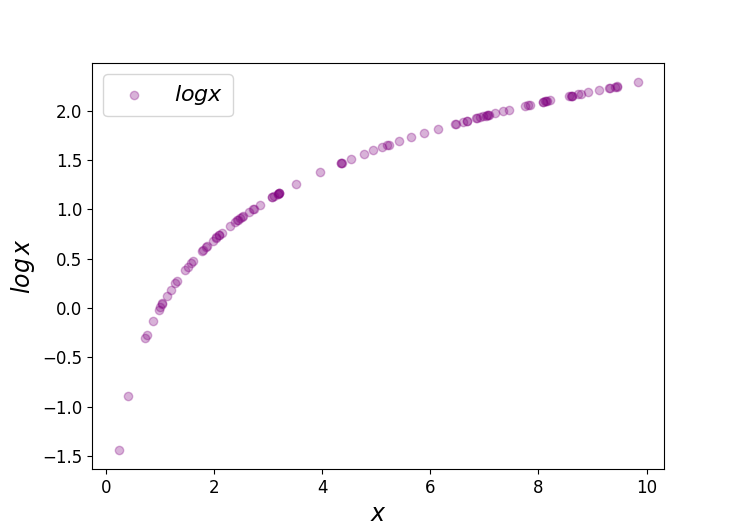



Logit Of Logistic Regression Understanding The Fundamentals By Saptashwa Bhattacharyya Towards Data Science



Logistic Regression
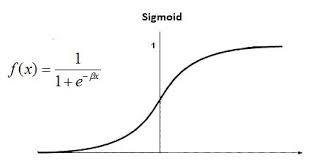



Advantages And Disadvantages Of Logistic Regression Geeksforgeeks



Logistic Regression Calculating A Probability
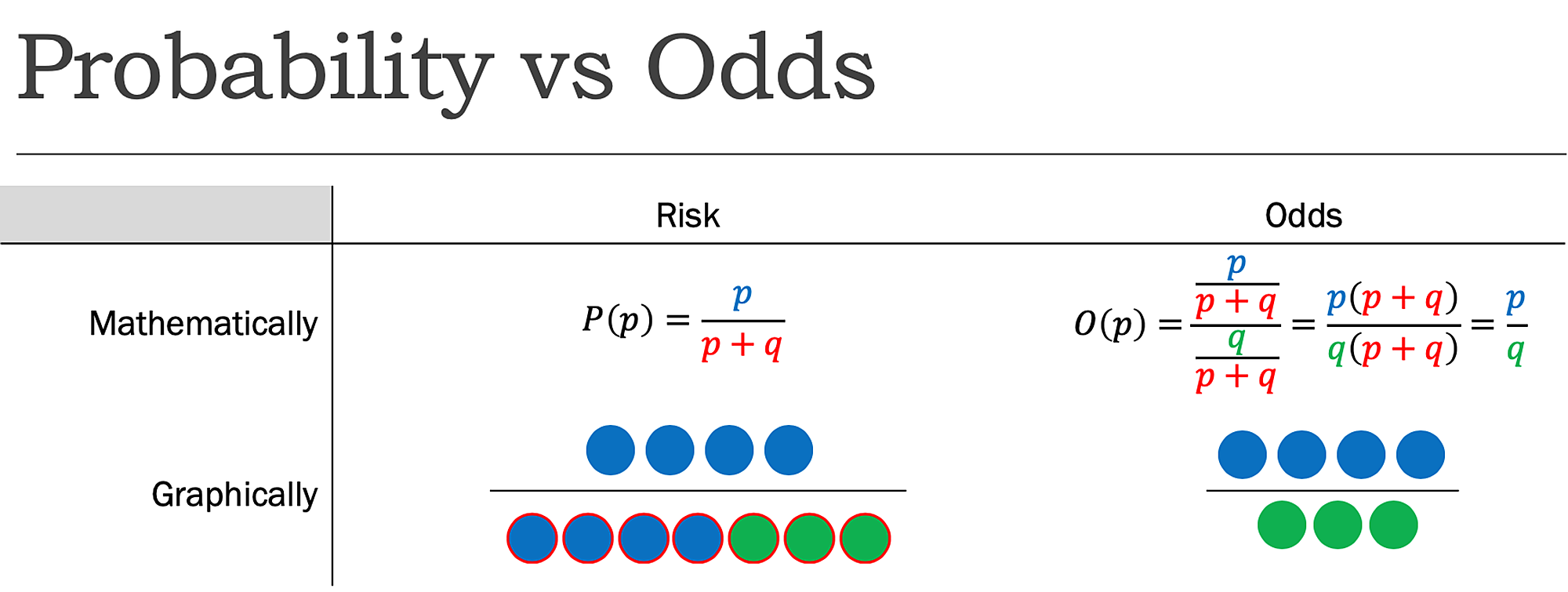



Cureus What S The Risk Differentiating Risk Ratios Odds Ratios And Hazard Ratios



Obtaining And Interpreting Odds Ratios For Interaction Terms In Jmp



Logistic Regression Wikipedia



1



Ctspedia Ctspedia Oddsterm
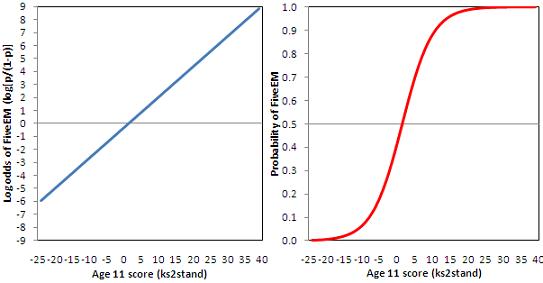



4 5 Interpreting Logistic Equations
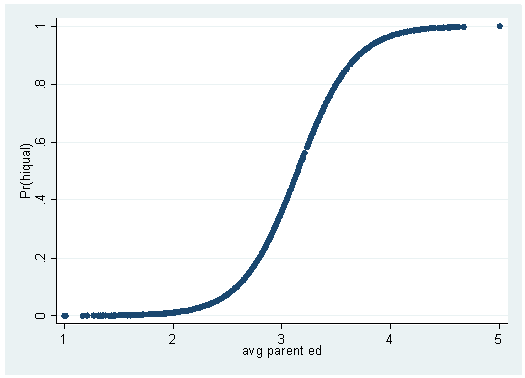



Logistic Regression With Stata Chapter 1 Introduction To Logistic Regression With Stata




4 2 Logistic Regression Interpretable Machine Learning



Q Tbn And9gctxz8owky Sul84xtk4ggzacxwhkmhguhlxwyjj9avufagdrhwm Usqp Cau



Logistic Regression Wikipedia



1
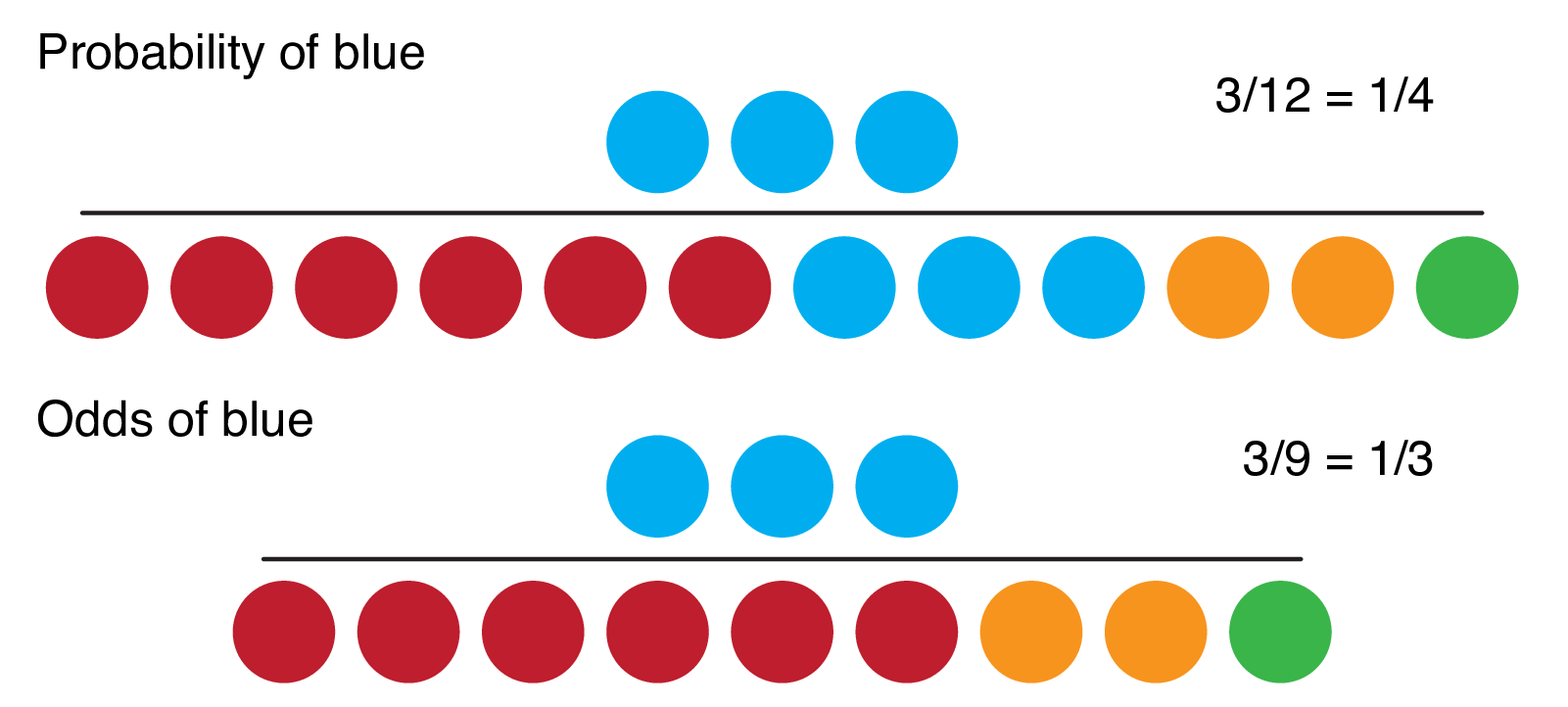



9 2 Binary Logistic Regression R For Health Data Science




Measures Of Association Log Odds Moore Statistics Consulting Llc




Logistic Regression The Journey From Odds To Log Odds To Mle To Woe To Let S See Where It Ends By Rutvij Lingras Analytics Vidhya Medium
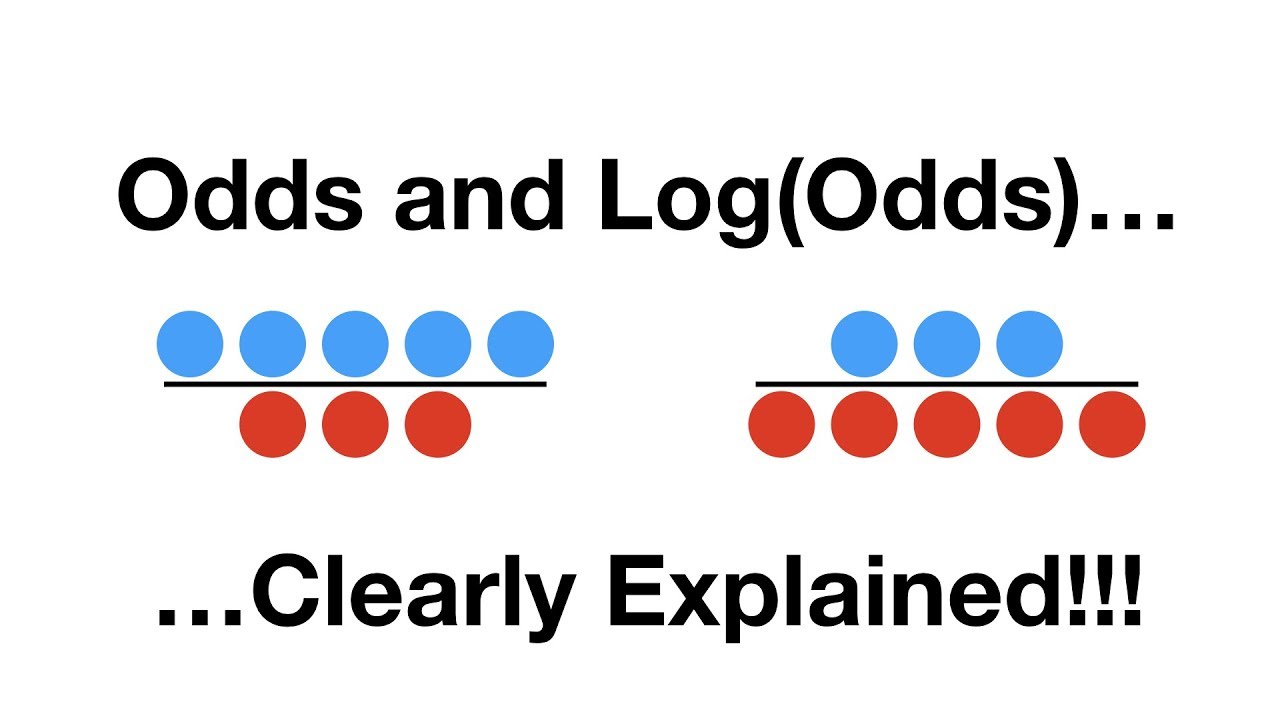



Statquest Odds And Log Odds Clearly Explained Youtube




Logit Of Logistic Regression Understanding The Fundamentals By Saptashwa Bhattacharyya Towards Data Science
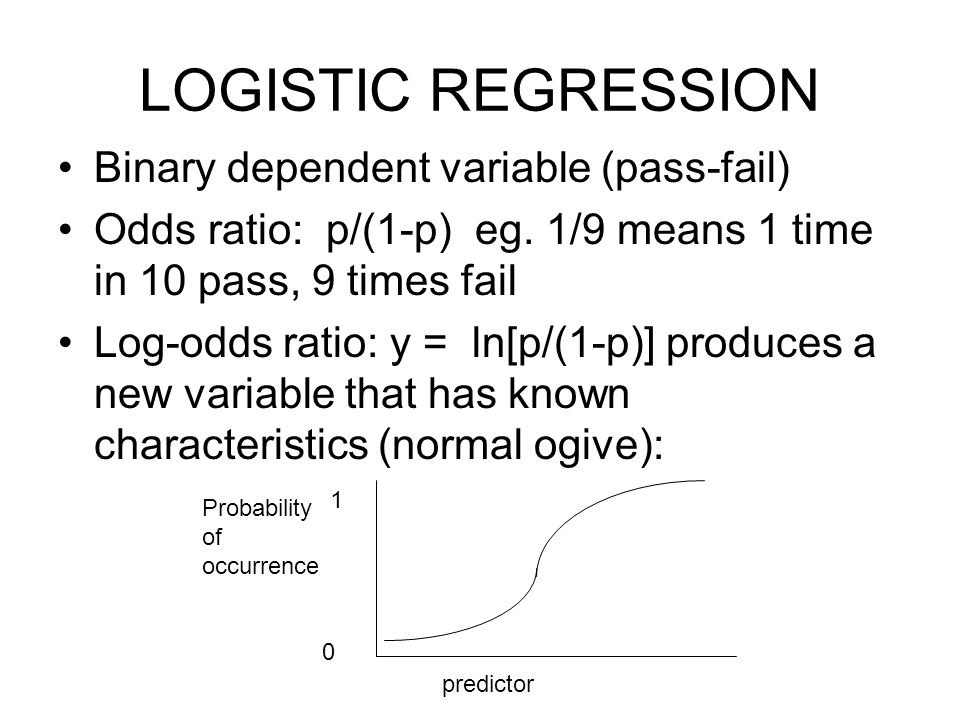



Logistic Regression Binary Dependent Variable Pass Fail Odds Ratio P 1 P Eg 1 9 Means 1 Time In 10 Pass 9 Times Fail Log Odds Ratio Y Ln P 1 P Ppt Download




Logistic Regression Estimates Odds Ratios Of The Probability Of Download Table




Keep Calm And Learn Multilevel Logistic Modeling A Simplified Three Step Procedure Using Stata R Mplus And Spss




Interpreting The Impact Size Of Logistic Regression Coefficients By Ying Ma Ro Data Team Blog Medium
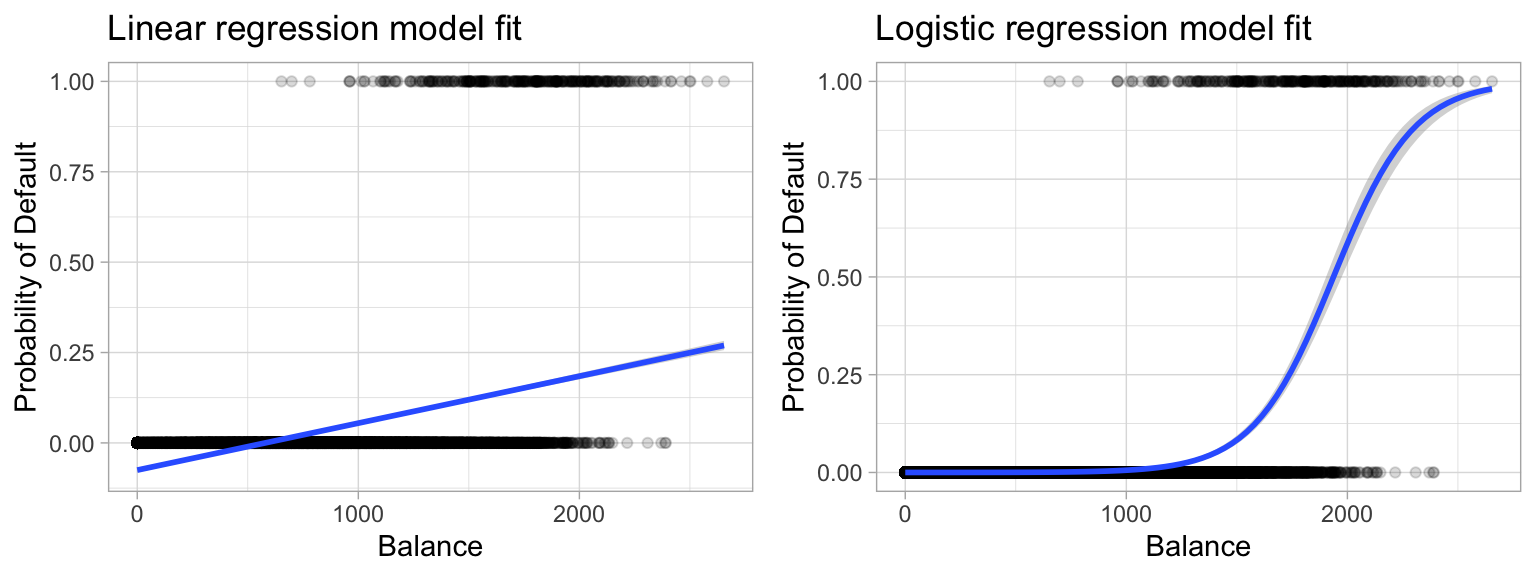



Chapter 5 Logistic Regression Hands On Machine Learning With R




Linear Vs Logistic Probability Models Which Is Better And When Statistical Horizons
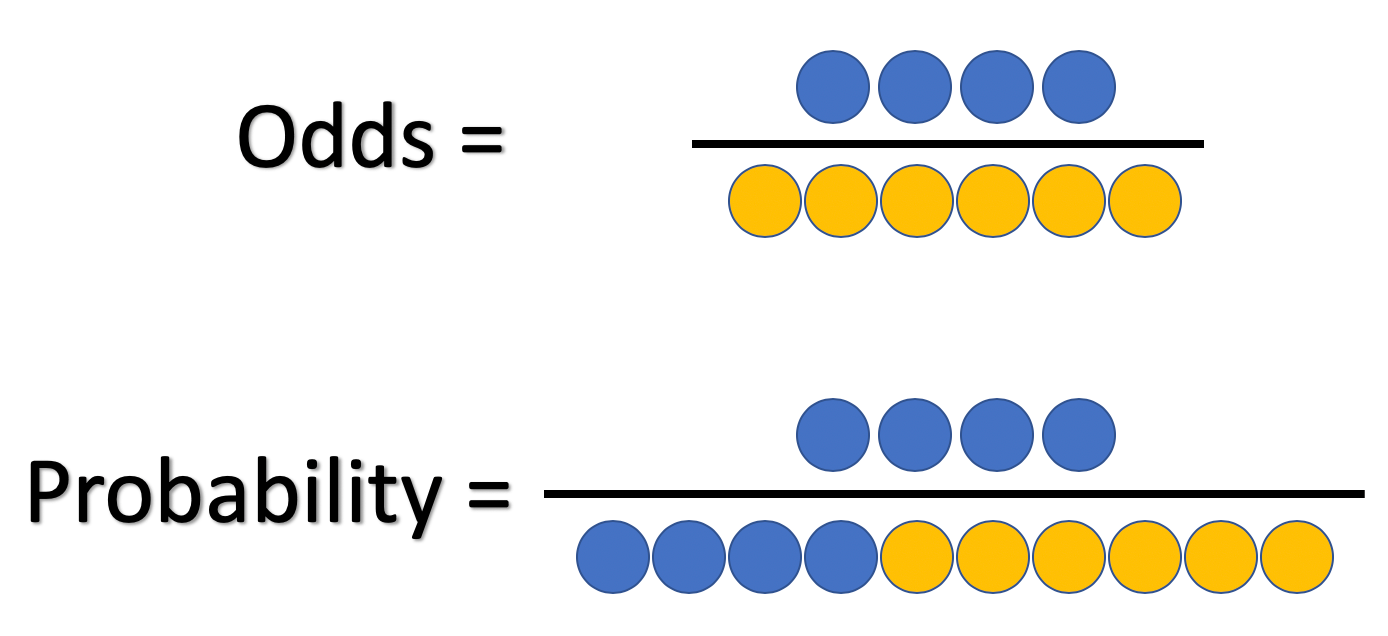



What And Why Of Log Odds What Are Log Odds And Why Are They By Piyush Agarwal Towards Data Science
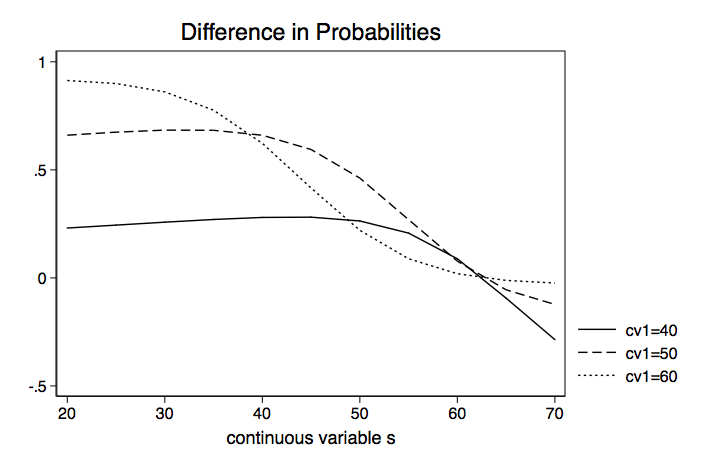



Deciphering Interactions In Logistic Regression




Odds Ratio The Odds Ratio Is Used To Find The By Analyttica Datalab Medium
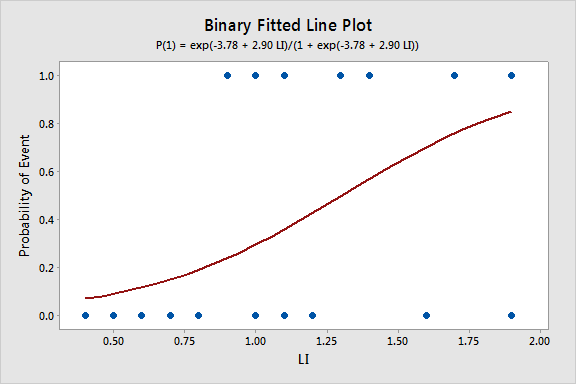



12 1 Logistic Regression Stat 462
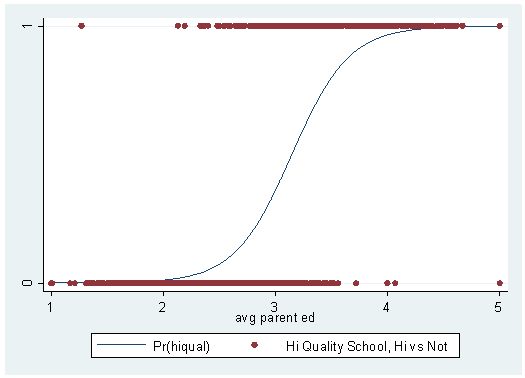



Logistic Regression With Stata Chapter 1 Introduction To Logistic Regression With Stata



Multivariable Logistic Regression Results A Forest Plot Showing The Download Scientific Diagram



Logistic Regression
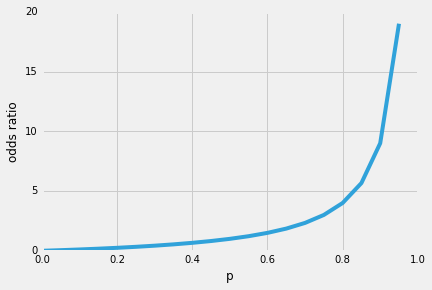



Logistic Regression Why Sigmoid Function
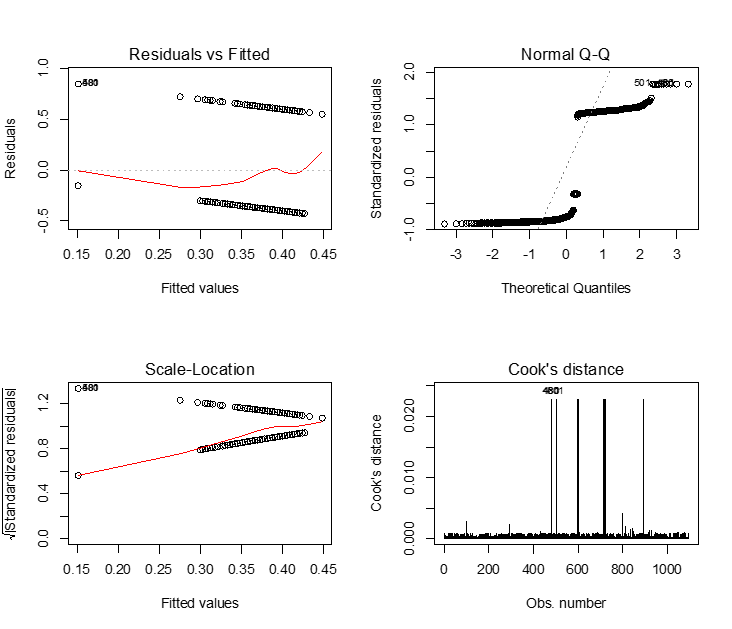



Logistic Regression



Logistic Regression
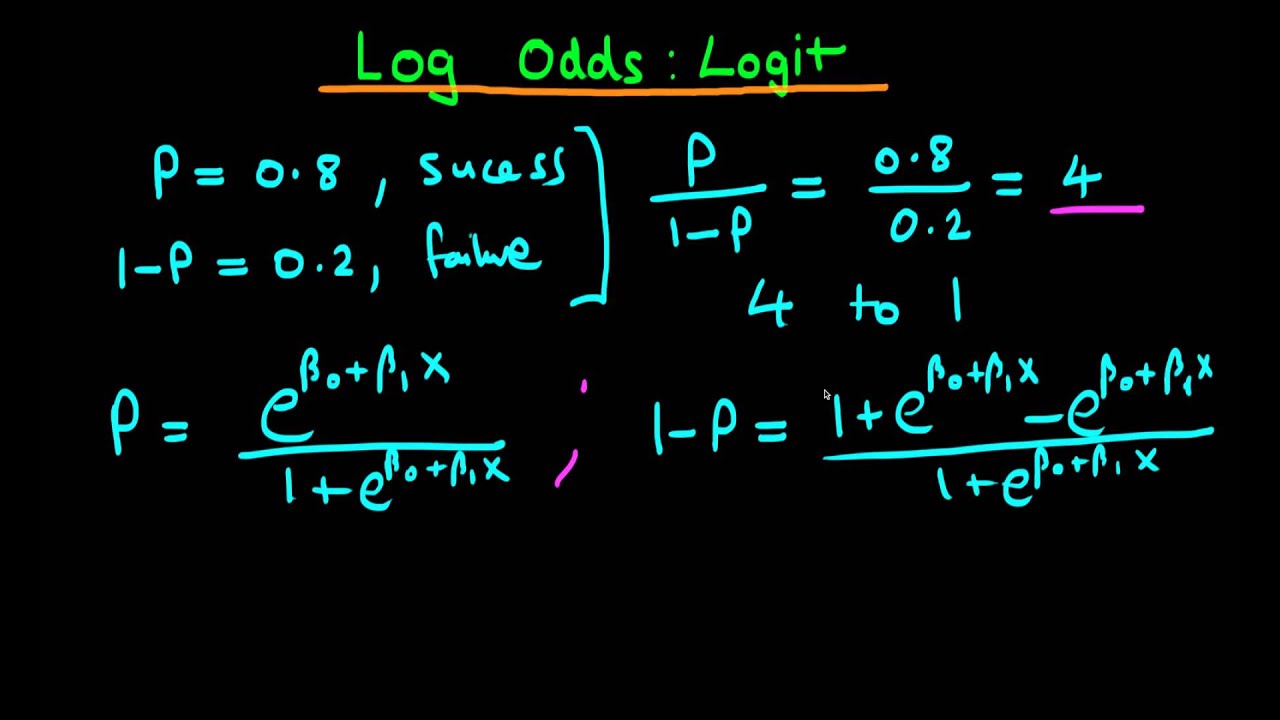



Log Odds Interpretation Of Logistic Regression Youtube




Binary Logistic Regression With Odds Ratios Calculated For The Download Table
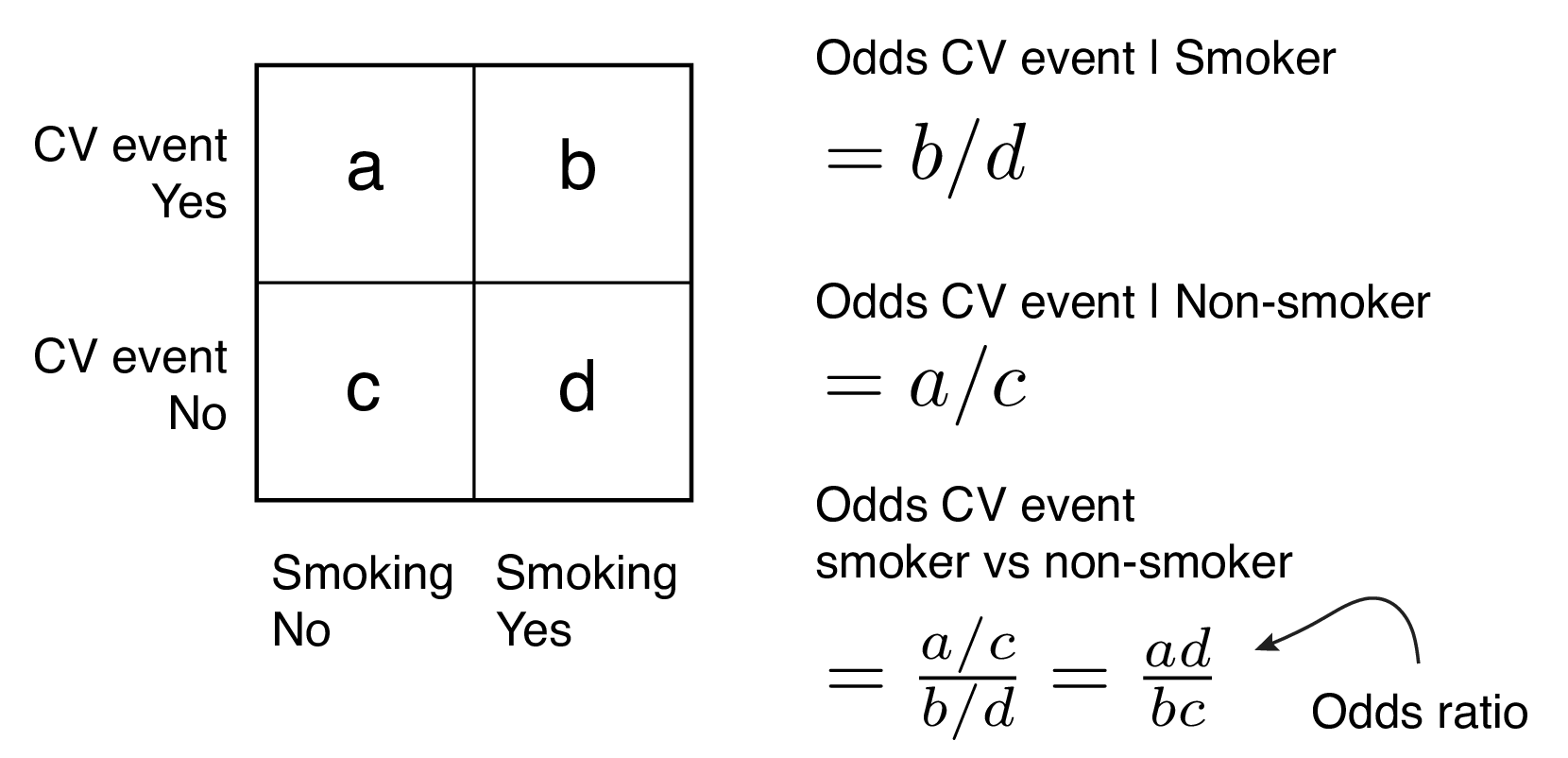



9 2 Binary Logistic Regression R For Health Data Science
0 件のコメント:
コメントを投稿